To many people new to user research, some clients included, the difference between quantitative and qualitative research seems simple — and comes down to large vs. small sample sizes and the presence vs. absence of numbers in the data. In the context of gaining the greatest possible learning, this oversimplified understanding often materializes in a question like, “Can’t we just run a survey?”
The answer is yes. You can always just run a survey. But, the real question is whether you should. Qualitative (qual) and quantitative (quant) research methods are not just different in the number of research participants; they present wildly different approaches to understanding human behavior and gaining insight. Nuances at play here come down to a foundational question of intent:
Do you want to uncover and generate a new opportunity for innovation?Or: Do you want to evaluate an already existing idea or assumption?
Based on where you are on your design adventure, you can make a call on which of the two — qual or quant — or even the sequence in which both may be applied, would be most beneficial to your current need. It is useful to realize that hearing from a large sample of respondents does not necessarily make research more sound. In fact, the scale achieved by quant research—through polls, surveys, large group sessions, or questionnaires—comes at the cost of tolerance for deviation. Deviation, or simply put, following a human curiosity to go off-book and deepen our understanding of why someone said something, is a luxury a survey cannot afford. And this intentional meandering, or ability to dig deeper into an unexpected topic that came up during an observation, intercept, contextual interview, shadowing session, or walk-along, is where qual research really shines.
Once you understand the contexts in which qualitative and quantitative methods triumph, it becomes clear that both categories of research are highly effective at doing what they do best.

For Generative Research, Use Qualitative Methods
At Highland, we tend to find ourselves doing qual research more than quant research. This is in some part because we conduct Design Research to uncover new opportunities for our clients. By understanding the motivations behind users’ behavior, we gain deep insight into their daily lives, impulses, and decisions, and articulate insights around users’ expressed and unexpressed needs. Knowing when to drift from the research plan—to ask about the anomaly in the room or the unexpected artifact on the wall—is crucial in this method. Only by adapting our questions based on what we see, hear, or learn can we generate new understanding of users and conceive solutions that are relevant and effective at addressing their real, unmet needs.
In other words, a lot of our work tends to be generative in nature. Generative research is all about gaining new understanding about user behavior that you would not have been able to predict or assume, identifying better questions to ask, and new territories to explore. It requires that the researcher show up with few, or no assumptions and hypotheses. This is exactly what qualitative methods are for: Show up. Bring only your curiosity. And be prepared to pay attention.
Quant methods, on the other hand, tend to be deeply incompatible with generative approaches. To design a survey, a researcher must make assumptions about the best questions to ask and pre-populate multiple choice answers to enable easy analysis after the close of the survey. Without even meeting or observing users, the researcher has already quantified their curiosity around users and structured the answers that they expect to hear. While open-ended questions or choices are technically possible in a survey, they infinitely complicate analysis methods and tend to be minimized for simplicity. This approach gravely limits the possibility of the researcher stumbling upon unknown or unexpected paradigms, counter to the philosophy of generative research.
Example: The Power of Qualitative Research in Generating New Understanding
On a recent project, when tasked with learning about and designing for a navigation experience, the team accidentally encountered new learning. In conducting intercept interviews in the context, we realized that the more we asked about navigation, the more users talked about the purpose behind the visit, alluding to the beauty and serenity of the space. Unsure of where this was going, we kept up our curiosity and continued the conversation. It became clear that in redesigning a navigation experience, we were not only addressing the functional need for directionality, sightlines, and accessibility, but also delivering against a more valuable need: boosting the sense of place.
Had we not followed users down the conversation trail in a generative research mode, we would have never encountered the bigger opportunity for the project. Any kind of survey or poll would have stayed within the functional sphere; we simply would not have known to ask about the immersive qualities of the space that motivate users to visit. We didn’t know that we didn’t know.
For Evaluative Research, Choose Carefully
So if quant methods are incompatible with generative research, what are they compatible with?
Quant methods are effective in evaluative research, not generative research. Evaluative research requires the researcher to have something to show or explain to users, and the intent of the research is to seek feedback or an evaluation. An A/B test, where the researcher shows two options and asks a large sample size to choose between the two is a common example of quantitative evaluative research.
Such quant evaluations are beneficial in gaining confidence in expanding to a new market or before the launch of a new product. Keep in mind is that quant evaluative research works well only when an opportunity or solution already exists. The assumption here is that an unmet need has been uncovered, identified as feasible and viable, and been designed for.
Qual methods can also be deployed for evaluative research. Qual research is — by design — flexible across generative and evaluative purposes, and consistently allows for greater context and user understanding. If during evaluative research, for example, you want to go beyond whether or not users will be attracted to a solution to the more ambiguous territory of why and why not, you might be seeking a qual approach. They allow for a deeper dive into fit with user context, flow with adjacent tasks, and behavioral tendencies. This way, you will understand nuance beyond just a yes or no relating to adoption of a presented solution.
Example: How Qual Methods Offer More Nuance During Evaluative Research
At times, that nuance is everything. Like on a recent client project: having uncovered unmet needs and prototyped possible solutions, our team wanted to understand desirability and fit with users. We could have done this evaluative learning through a quant survey, perhaps leveraging a MaxDiff methodology to discern which of the potential solutions may be most desirable to a large sample of users. But we’re glad we didn’t, instead choosing a qual approach to understand the deeper why behind responses. What we uncovered surprised us greatly: when presented with a range of relevant, helpful solutions delivering on unmet needs, users tended to ignore those that they didn’t effortlessly comprehend at first glance.
This means that even though a potential solution may have resonated with their desires — possibly more favorably than others — it would not have been adopted because a quick and simple understanding was missing. Imagine our direction if we had gone down the quant route: in the absence of this behavioral understanding, we may have rejected valuable, needed solutions because of a fixable issue with design communication. Thanks to qual research, we avoided what could have been a big mistake: developing a less valuable solution because users did not as easily understand other, more valuable options at first.
With evaluative research, quant methods are an option for learning. But using qual methods for an evaluative purpose can provide both an evaluation of what you have, as well as additional, adjacent understanding that might make all the difference.
What People Say ≠ What They Do
Perhaps most importantly, qual and quant methods differ in their effectiveness at learning from different levels of a participant’s self-awareness. We know from behavioral science that we humans don’t know ourselves as well as we think we do. We’re pretty bad at accurately describing our own motivations, behaviors, and decision-making.
Qual research accommodates this lack of human self-awareness to understand the real behavior that the human in question might not even be aware of. To do this, a skilled qual researcher avoids an interview question like:
- “What makes you decide on something?”
- And asks one like this instead:
- “Show me how you went about making this decision” and observes the user as they demonstrate a real-life situation.
This way, the focus is on what users do, not what they say. Such probing is a much more dependable way of uncovering behavior, emotion, and needs. Qual methods like this allow a researcher to understand how participants see themselves, and during synthesis, compare/contrast that with the actual behavior a researcher observes. Even though we may hear a lot of wants during a research session, observation and synthesis point us directly toward needs.
On the other hand, quant methods lack in clearly distinguishing between what a participant says and what a participant actually does. With quant, a researcher cannot demand proof of users’ claims, and depending on how questions are worded, might be incentivizing a preferred or morally superior response. These shortcomings, along with the lack of observation in quant methods, makes it difficult to know: did this user respond based on what is true about their behavior, or what they falsely believe to be true, or in certain cases, want it to be true because it looks better?
Here’s an example that illustrates this: we worked with a client recently that believed they understood their target market based on quant surveys. The client presented their target market as diligent and intentional decision-makers, people who would work to gain a robust understanding of any topic before they made decisions. When we conducted subsequent qual research to learn more, we found that our clients had made assumptions about user behavior based on attitudinal and demographic data. While it was true that users viewed themselves as intentional decision-makers, their actual behavior in day-to-day life didn’t correlate. These users tended to rely on gut-checks and emotion over research and due-diligence — distinct from meticulous and intentional decision-making — because of common constraints like lack of time, lack of energy, and plain old procrastination.
Qual methods are the only effective option for digging deeper than user perceptions to get at unmet user needs. If you want to know how people perceive themselves and how those perceptions may differ from real behaviors, then a qual approach is the best fit for you. If you just quickly want to know how many people prefer roast beef over turkey, without knowing why or why not — then qual is just going overboard, and you might as well conduct a survey and save the effort.
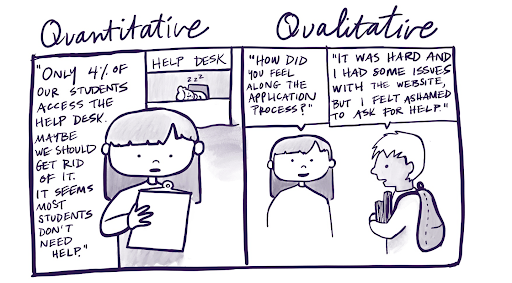
Choosing a Process: Analysis or Synthesis?
Qual and quant methods also differ in terms of the process they require to make sense of research findings.
With quant methods, findings are direct and measurable and come down to quantities: i.e., how many people answered x instead of y. The most demanding thinking happens at the front-end when researchers are preparing the survey, poll, or questionnaire. This is when researchers think of the questions to ask, how to ask them, how to allow users to respond, and how data will be collected and processed. Once the research is complete, the task is centered around analyzing responses — aggregating what was received, and presenting it through visuals.
With qual research, questions asked during the research are important, but the real work happens after the fieldwork is complete, through a process called synthesis. To discern deep understanding from qual methods, the researcher must become intimately familiar with every user’s responses and the context in which they were mentioned — impossible in a quant approach — and cluster observations based on patterns of motivation. These clusters, based on common needs across behavior, lead research teams to aha moments — or insights — that direct us toward design opportunities.
The synthesis process is interpretive, time-consuming, taxing, and often the most challenging part of the qual process. But synthesizing is worth the soul-searching because it takes us to new places, crystallizing new understanding that may be hidden in plain sight. For example, on a recent internal project, analyzing a research track helped us understand how much users were leaning toward a hypothesis. But by synthesizing the same information, we realized that larger forces were at play beyond our hypothesis, redirecting the project and our attention toward underlying emotions to accidentally define distinct — and unexpected — user personas.
Unlike synthesis, which requires interpretation, analysis is about consolidated—and uninterpreted—reporting. But the real question while discerning between the two is: is the researcher simply wanting to prove or disprove what was hypothesized, or is the researcher open to the possibility of unrealized meaning gained at the end of synthesis?
To Use Quant Responsibly, Start with Qual
Because of the mostly generative, ambiguity-embracing, discovery-based research we do at Highland, we frequently choose qual methods over quant approaches. We do appreciate, however, that at times, quant methods are incredibly helpful, and are sometimes required to make decisions toward or away from a solution. When resources are limited, and a client can only spend so much money or time on an existing product, quant tools like top tasks and benchmarking are vital for determining which features should be prioritized and which usability problems should be addressed first.
With that said, we would never recommend quant approaches as a Design Research methodology. To truly deliver on Design Thinking — a codified practice embracing empathy, synthesis, systemic thinking, and iterative prototyping — requires taking a qual-first approach. By first uncovering new dimensions behind human motivation and opportunities hidden in user behavior through a qual approach, we are in a better position to subsequently evaluate ideas and solutions qualitatively or quantitatively.
Taking a quant-first approach — defining market opportunities, needs, or user segments based on inflexible research that does not allow for behavioral nuance — may result in starting in the wrong place. A small mistake made at the outset of quant research can have an outsized and undesirable impact later on.
Example: Quant without Qual is a Recipe for Mistakes and Garbage Data
An example of such a mistake came to light recently on a client project. Before collaborating with Highland, a client strongly believed their customers fit a particular profile due to responses to a multiple-choice question used in their survey. They were so sure about the meaning and associated assumptions with this data that when our qual research offered up insights that contradicted this belief, the clients questioned the very validity of the qual research.
When we looked at the client’s original direction-setting quant survey to better understand the situation, we saw that the question was written in a way that did not reflect reality for respondents and did not provide them an opportunity to say so. Instead, respondents only had access to multiple choice responses, and when those responses came in and were analyzed, the numbers showed that x number of people answered in a certain way. Neither the responses nor the analysis revealed the truth of the matter: that the question was incorrectly written, and the responses cannot be trusted.
With qual methods, a mistake of this magnitude — that leads to compounded mistakes later on — is less likely. In the same example, if qual methods were used instead of a survey, the mistaken assumption in the question would have been ferreted out much earlier on, simply by the confused look on users’ faces. The client wouldn’t have wasted resources conducting project-defining research only to inappropriately delegitimize later findings that didn’t support the mistaken belief. Even if your qual research includes questions that make incorrect assumptions, these are quickly defeated with rich, valuable, contextual information resulting from talking to and observing people.
Complementary, Not Competitive Methods
The key is to use both qual and quant methods for what they do best. Qual research is poised to understand something in depth, uncover things you don’t know you don’t know, and get a view into real human behavior and the ways people think. Quant methods are for scaling those findings — as with market research — to answer questions like: how many people encounter the same issue? How many of those are seeking a solution? And, How many resonate with our solution ideas?
The things that are easiest to measure are not necessarily the most valuable. While quant findings can provide the comfort that comes from metrics and large sample sizes, perhaps what you really need is the deeper meaning that comes from rigorous, interpretative, and motivation-based synthesis. Just ask yourself: “Should we really be running a survey? Can’t we just do some qual exploration first?"